Players that churn from your game are a wasted investment, resulting in lost income and lost data. This guide will show you how analysing players for churn can help you reduce the number of people that bail on your game by implementing strategies to engage them before it is too late.
Churn is a big deal, especially for mobile games where the attention span is short and players can go from “this game is cool” to uninstalling it a few days later, or just forgetting about it.
Many strategies around churn reduction are around Live ops, events, dynamic storefront and campaigns, daily or weekly rewards and other strategies to incentivise the player to stick with the game.
But this is not what I want to talk about today, these strategies are there for all players, they benefit all the player base and you often don’t have any adjustments based on user-level engagement metrics.
In this guide, I want to show you how you can go from identifying likely-to-churn players to engaging with them to make sure they stick to the game.
Before I jump in, please be aware of the potential impact. You won’t get them all back but a 3~5% uplift goes along when you have a holistic view of your cohorts. Additionally, some experiments may help you tune the experience for the whole player base and will benefit your other metrics.
Using built-in features to identify churn
Some tools will actually have built-in features that allow you to identify churning users. Always make sure to understand how the algorithms for scoring are built: what you are looking for are engagement signals, more than inactivity triggers.
What I mean is that tools showing you users that are likely to churn but are still active will allow you to pick up the problem earlier vs tools that will warn you when users have been inactive for a certain amount of time.
Knowing exactly what is used to score users is not possible, but you can assume it will be a combination of avg number of events per session, session duration variation and session frequency variation.
A few platforms that use similar approaches for churn prediction segmentation:

Using analytics tool to calculate churn
I’ve worked on two different churn prediction projects that both failed. Churn prediction is a difficult topic and identifying users that are likely to churn does not always use the same strategy for all games.
But there are proxies you can leverage to identify users that are likely to change or at least that are not enough engaged when compared to your core audience.
Early churn (users installing the game, then likely to churn right away)
- Short term inactivity: Easy one, just detecting that users are inactive for 3/7/14 days gives you a really good clue that users may be dropping on soon (or are already dropping off)
- Features adoption: If you believe some features are mandatory for players to experience the core value of your game, identifying that users did not experiment with some features even after their 5th session is a good signal (e.g. player didn’t upgrade a card since they did it in the tutorial)
- Social link: This is similar to features adoption but it’s linked to all social interactions like adding a friend or joining a clan. Players that are playing with their friends or growing in a community will likely have a better stickiness. This also means that early users missing these features are likely to drop earlier from the game.
- Defeat frequency/streak: It’s not fun to lose in a game, if you can detect early that the player is struggling, it’s a good trigger to help them get better.
Mid-level churn (Engaged players, a few weeks since install)
- Short term inactivity: Same as early churn, fairly easy to detect
- Session frequency variation: More difficult to identify but can be really powerful when it’s done right. Identifying when the avg session frequency per day / per week is decreasing (e.g. last 3 days on last 14 days) will give you some guidance on how players are sticking with the game and if it’s likely they may drop off in the near future.
- Session duration variation: We’re not looking at session duration but how the avg session duration for the last couple of sessions is different from the avg session duration on user level. This allows us to identify, the same way the session frequency can give signals, if the player activity is lower than usual.
- Session density: This is an approach that can be leveraged if you have a sophisticated game loop with multiple progress touch points (e.g.: players need to do their dailies, weeklies and upgrade some items). This is also a tricky one but you can potentially define a weight for each action based on how you believe it correlates with long term engagement (e.g.: playing a game is 1, finishing dailies is 2, fighting a friend is 3), then give a score for each session, then analyse the avg density per session for all players. This is extremely complex to set up but probably gives the biggest granularity in understanding the core users moving to likely to churn (avg density from 3 to 0.5 is alarming).
How to integrate these approaches
If you don’t have capabilities, start simple: for early churn, you can use all the recommendations with a basic analytics tool. For mid-level churn, just use inactivity or leverage a tool to give you additional signals. Some free options for platforms would be Firebase and Amplitude
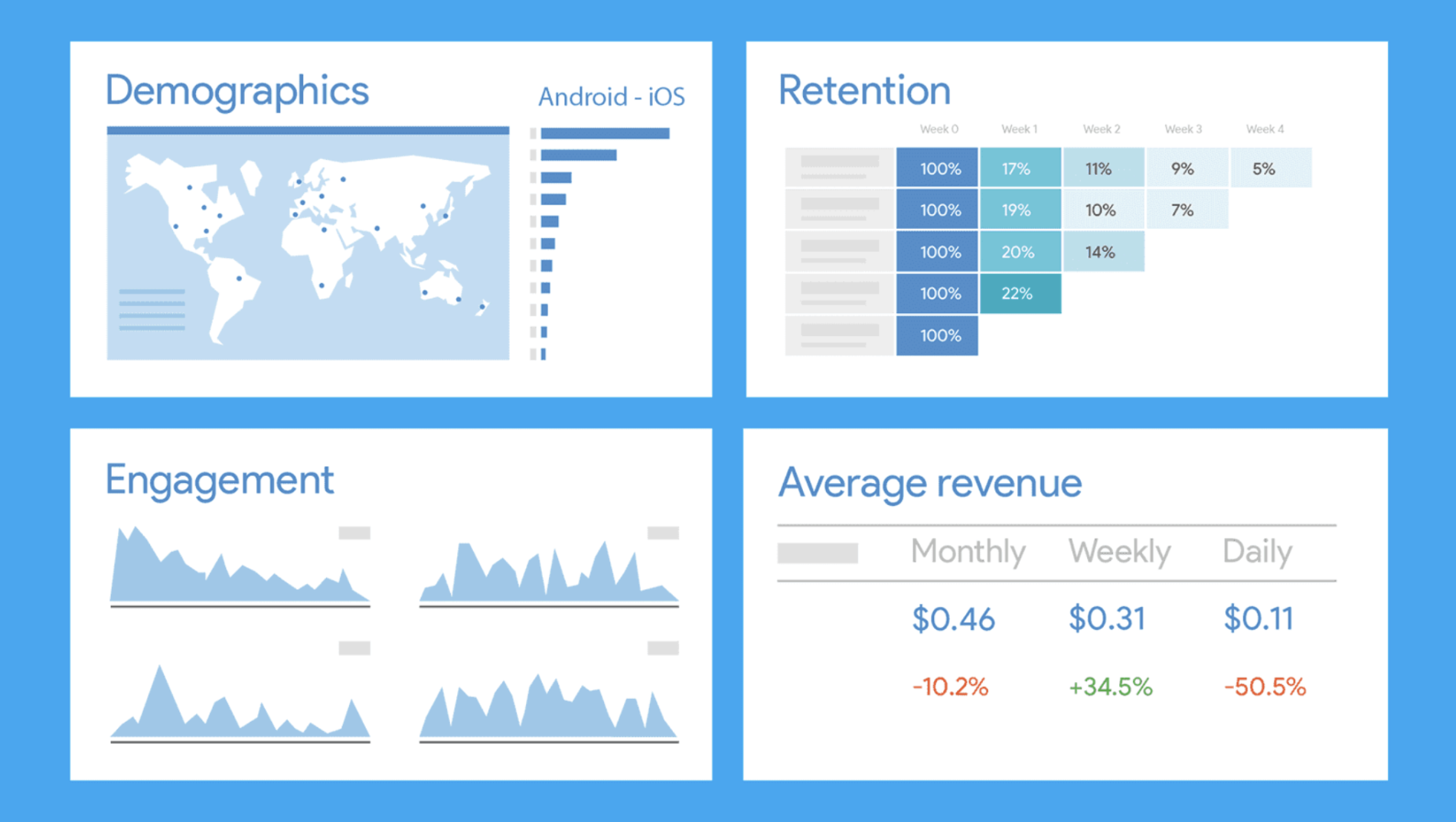
Conclusion
The aim of churn reduction is to make sure your game doesn't die two weeks after a player instals it. You want the player to return regularly, collect rewards and unlock new stages/levels. Churn can be more expensive than acquisition in mobile games as you have paid for user acquisition already (advertising costs, a big part of UA costs, goes towards acquiring users) but you still need to invest in keeping them around.
In this guide I've shown you how several game analytics tools can help you reduce churn. There are many ways to reduce it and to optimise your game based on what you define as 'right'. I strongly suggest spending more time understanding your players before reinforcing a strategy that has no relation to the way they play. Be careful in your engagement while digging deeper into key issues and be ready to work slowly with the data you have.
In addition to that, here you find 10 experiments to reduce churn in your game.
Written by: Kevin Bravo